Deepseek AI vs ChatGPT
Introduction
The world of artificial intelligence is evolving rapidly, and new AI models frequently challenge existing giants. Deepseek AI vs ChatGPT has become a hot topic in recent discussions, with Deepseek AI making waves by surpassing ChatGPT in just a few days. How did this happen? What makes Deepseek AI superior? Let’s explore this battle of AI giants in detail
Understanding Deepseek AI and ChatGPT
What is Deepseek AI?
Deepseek AI is a next-generation AI language model designed to improve upon existing AI capabilities. It focuses on enhanced real-time learning, superior contextual understanding, and faster processing. Its ability to dynamically update and learn from new data gives it an edge over traditional AI models.
What is ChatGPT?
ChatGPT, developed by OpenAI, is one of the most popular AI models used for conversational AI, content generation, and problem-solving. However, it relies on periodic updates, meaning it doesn’t learn in real time like Deepseek AI.
Key Features Comparison - Deepseek AI vs ChatGPT
Accuracy and Context Handling-Deepseek ai vs chatgpt
Deepseek AI surpasses ChatGPT in understanding complex queries and maintaining long-term conversation context, ensuring more relevant and precise responses.
Speed and Performance
Deepseek AI’s response time is significantly faster, making it more efficient for real-time applications.
Training Data and Model Size
While ChatGPT relies on pre-trained datasets, Deepseek AI continuously learns from real-world interactions, allowing for better accuracy over time.
Customization and Flexibility
Deepseek AI allows for greater personalization, making it more adaptable for businesses compared to ChatGPT’s standard responses.
Unlock Your Business Intelligence Potential with Power BI!
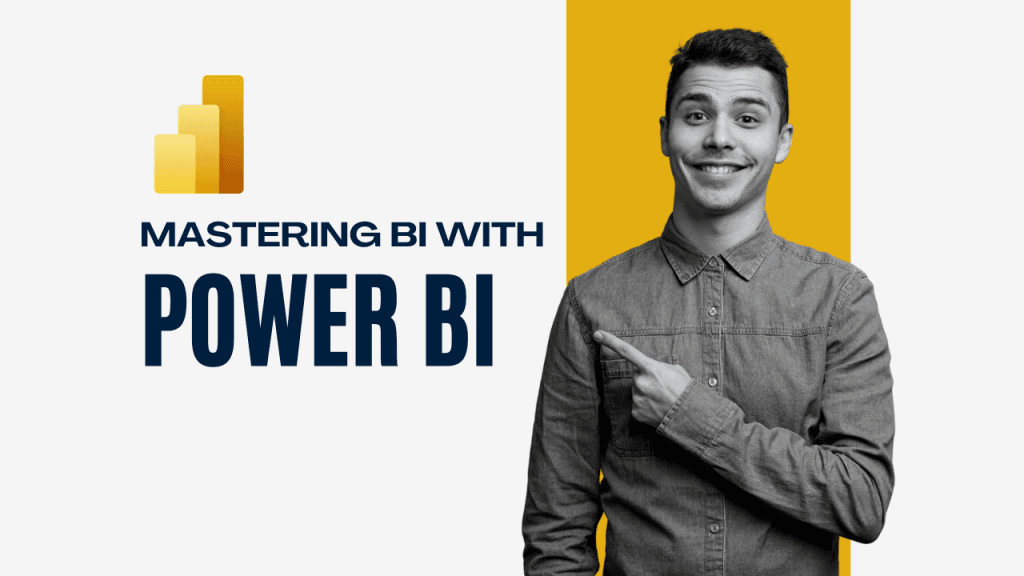
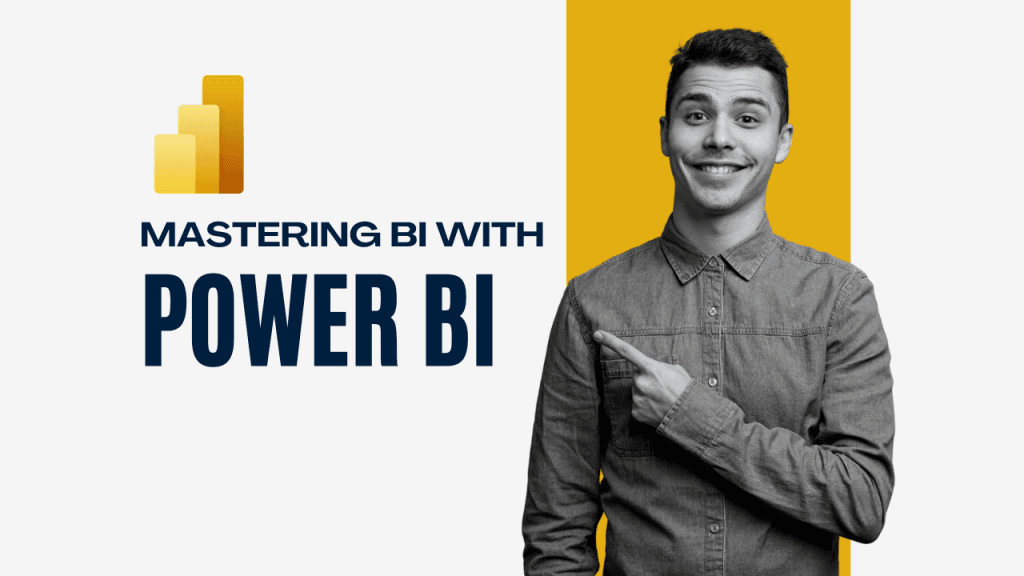
Unlock Your Business Intelligence Potential with Power BI!
How Deepseek AI Surpassed ChatGPT in Just a Few Days
Real-time Learning Capabilities – Deepseek ai vs Chatgpt
Deepseek AI’s biggest advantage is its ability to learn in real-time, unlike ChatGPT, which requires periodic retraining.
Advanced AI Algorithms
Using cutting-edge deep learning models, Deepseek AI optimizes responses faster and reduces errors.
Multilingual Support and Global Adoption
Deepseek AI’s wide-ranging language support has made it a go-to AI model for a global audience, surpassing ChatGPT in accessibility.
Pricing and Accessibility - Which One Offers Better Value?
Free vs Paid Plans – Deepseek Ai vs Chatgpt
Deepseek AI offers a more affordable pricing model, making it a cost-effective solution compared to ChatGPT’s premium features.
Business and Enterprise Solutions
Companies looking for AI-powered automation find Deepseek AI’s adaptable architecture more beneficial than ChatGPT’s rigid system.
Limitations of Deepseek AI and ChatGPT
Data Privacy Concerns – Deepseek ai vs chatgpt
Both AIs face scrutiny regarding user data security and ethical concerns.
AI Bias and Ethical Issues
Bias in AI responses is an ongoing challenge. While Deepseek AI has improved in this area, no model is entirely free from biases.
Future of AI: Will Deepseek AI Continue to Lead?
Expected Improvements
Both AI models will continue to evolve, but Deepseek AI’s dynamic learning approach gives it an edge.
Competition in AI Market
Other AI models are entering the competition, making the AI race more intense than ever.
Conclusion
Deepseek AI has successfully surpassed ChatGPT in just a few days due to its real-time learning, improved accuracy, and faster processing speed. However, the competition is far from over, and both AI models will continue to evolve.
Deepseek AI Tutorial
FAQs
Which AI is better, Deepseek AI vs ChatGPT?
Deepseek AI currently outperforms ChatGPT in speed, real-time learning, and accuracy.
Why did Deepseek AI gain popularity so fast?
Its real-time adaptability and improved contextual understanding gave it an immediate advantage.
Can businesses use Deepseek AI for automation?
Yes, Deepseek AI’s customizable features make it ideal for business automation.
Is ChatGPT still relevant despite Deepseek AI’s advancements?
Yes, ChatGPT remains a powerful AI tool, but Deepseek AI is pushing boundaries further.
How do Deepseek AI and ChatGPT handle real-time data?
Deepseek AI learns dynamically, while ChatGPT relies on periodic updates.
Unlock Your Business Intelligence Potential with Power BI!
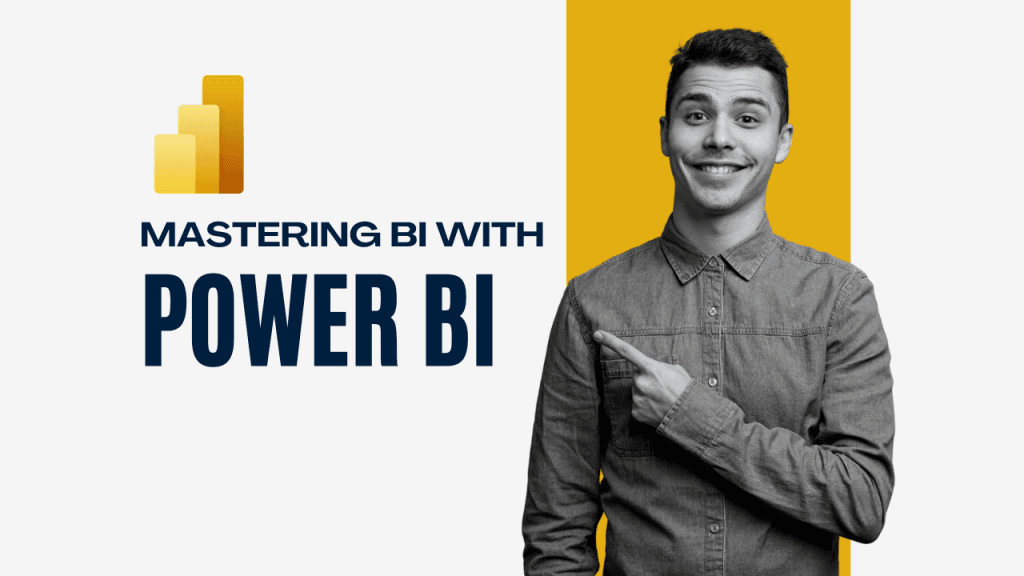
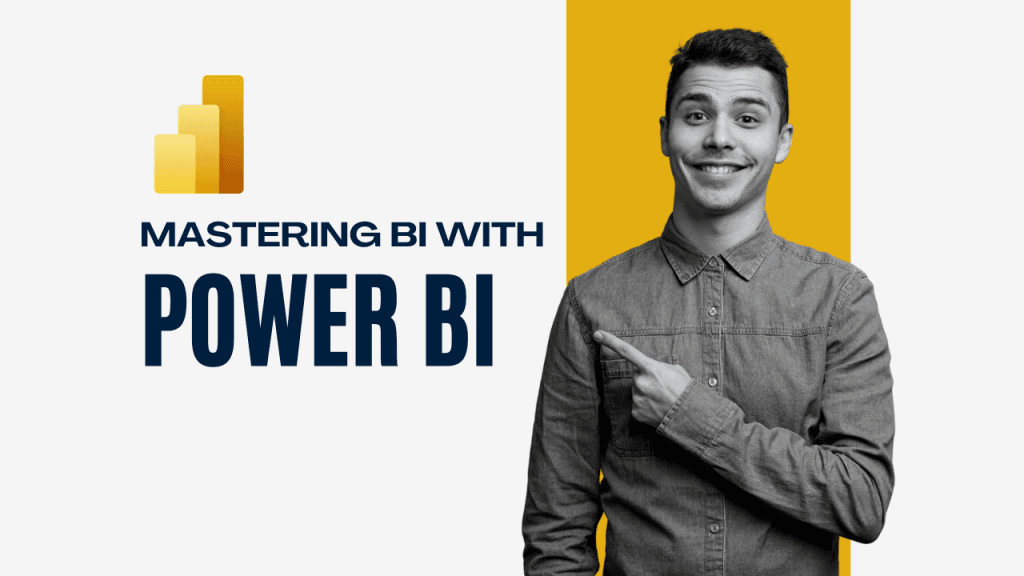