UNP Education
- 12 Sep, 2024
- 0 Comments
- 3 Mins Read
Is Artificial Intelligence and Data Science Engineering are Different
When it comes to technology two words that are most commonly used in parallel are Artificial Intelligence and data science engineering. Thus, it is also important to understand what the differences between the two are, although they are rather close in many aspects. These are reviews of do’s and don’ts which briefly explain engineering characteristics of AI and data science that will be discussed in this article.
Table of Contents
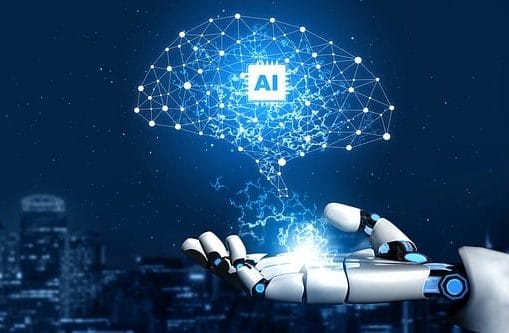
AI or Artificial Intelligence can be defined as the enactment of intelligent machineries to execute functions that are usually done by humans. They are meant to be programs that have the capacity to adapt in learning from data and then make a decision or a prediction. AI as a broad subject comprises of many disciplines among them are machine learning, natural language processing computer vision and robotics.
Ready to take your data analysis skills to the next level? Check out our comprehensive Python for Data Science Course!
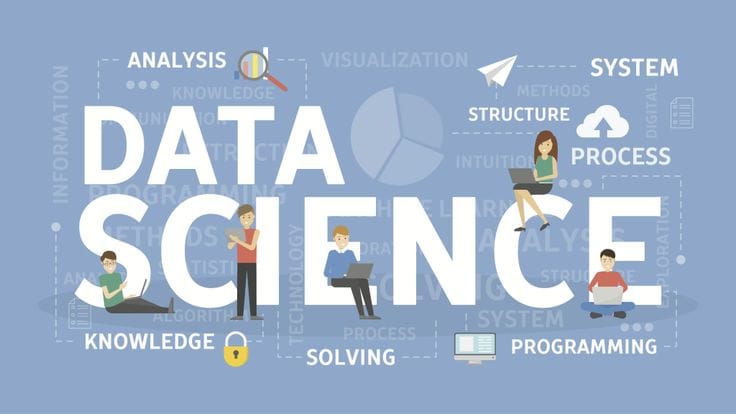
Data Science Engineering, on the other hand, is a field that deals with the identification of actionable patterns from large numbers. This job requires the application of statistical analysis, data mining, and programming skills to solve data sets problems. Analysts use equipment and software solutions to analyze the initial unprocessed data in order to extract significant information for the company.
Distinctions between AI and Data Science Engineering :
While, therefore, AI and data science engineering have several similarities in terms of their professional profiles, the two career paths are rather different.
1. Scope and Purpose: AI is the science of creating smart machine that are capable of functioning and making decisions like humans. – Data Science Engineering deals with the data management to arrive at useful conclusions and to implement the same for dealing with authentic issues.
2. Techniques and Methods: Discovered as machine learning, deep learning, and neural networks, AI makes it possible for systems to learn from datasets and predict or decide. – In Data Science Engineering, there are procedures such as statistical analysis, data visualization, and data mining with the primary intention of finding patterns, trends, and correlations in the data.
3. Applications: – AI is used in various fields such as, home assistants, self-driving cars, financial forgery detection, and recommendation engines. – Consequently, Data Science Engineering has numerous applications in the fields like Business Analytics, Predictive Modeling, Healthcare Analytics, and Predictive Analytics for Forecasting.
4. Emphasis on Data: AI strongly depends on data in order to train the models and increase the effectiveness of the employed systems. – Data Science Engineering deals with identifying data, acquiring the raw data, cleaning it and transforming the data into an understandable format and making it useful for some or the other decision making process.
5. Skill Sets: AI personnel need clinical knowledge of the algorithms, programming languages – Python and/or R, and skills in designing and training AI systems. – Data Science Engineers are individuals who work with data and to perform data analysis and have expertise in statistical analysis, data manipulation, data visualization and also have data knowledge so that they can make valuable insights from data .
Therefore, it can be summarized that although artificial intelligence and data science engineering are related and share close links, they were established with different aims and strategies. AI is about designing intelligent machines that can perform the tasks done by human beings on their own, while the data science engineering is about how to acquire a rich and useful information from available sources to conquer the existing problems. It is necessary to understand the differences of both fields when it comes to implementing and developing advanced technologies and innovations.
Ready to take your data analysis skills to the next level? Check out our comprehensive Python for Data Science Course!